Essays
What Is Realism in the Age of AI?
Photographs have long been manipulated or altered, but the rise in generative models gives new meaning to the idea of a “real” image.
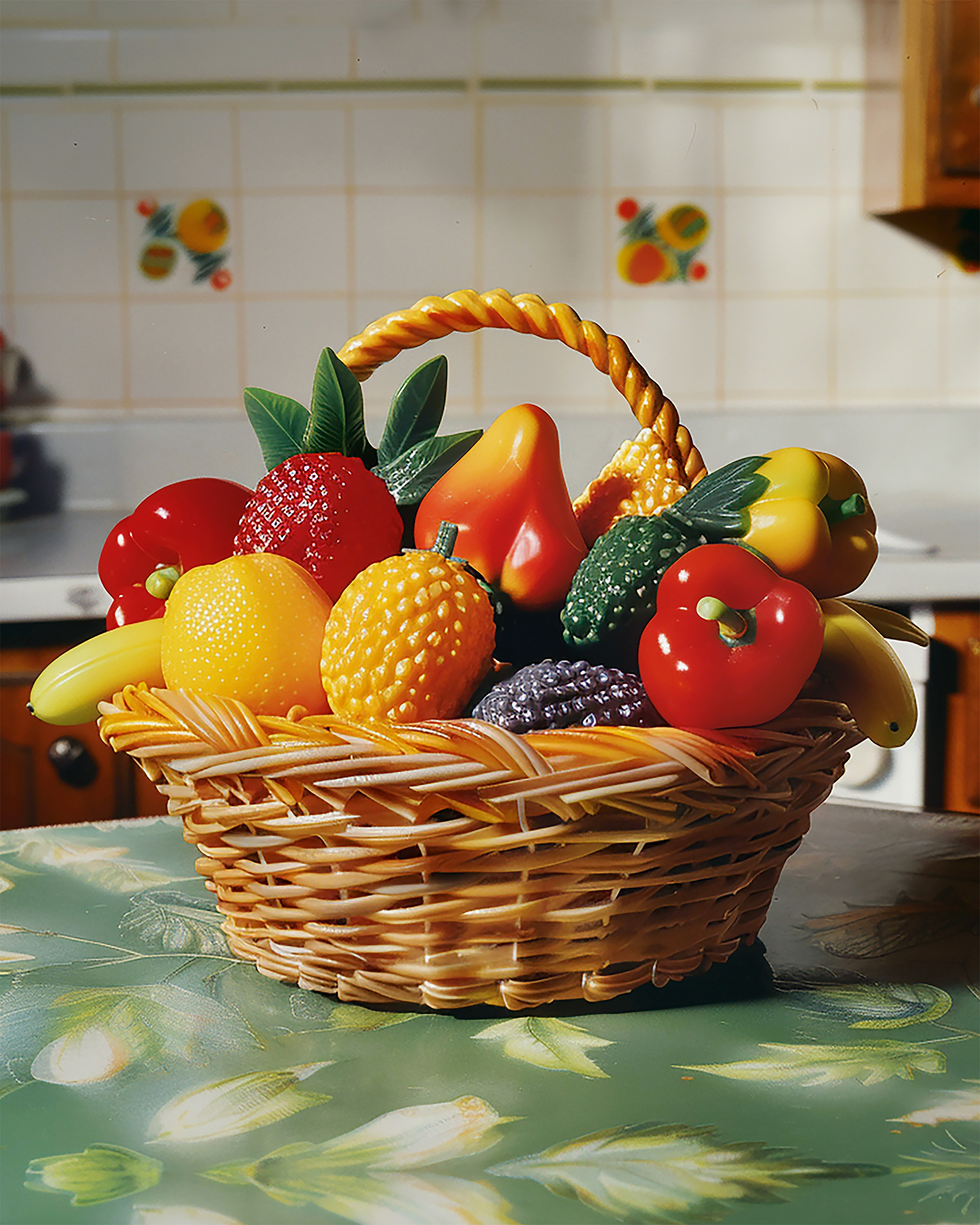
With the advent of generative models that can simulate photography and make images from math, it has become necessary to be more specific in describing images that are not manufactured using such procedures. But what to call them? The term “real photo” was used by several tech news sites in headlines this past summer in reference to Meta’s mislabeling of archival photographs as being “Made with AI.” “Real photo” seems intuitive enough but is misleading if not blatantly oxymoronic. Isn’t every photograph unreal to the extent that it is a representation and not something real, in and of itself? Doesn’t “real photo” remind us of how dubious it has always been to complacently assume that photography objectively documents reality? All the images in question on Meta’s platforms are made of digital code rather than light and chemicals on paper. Does that disqualify them all from realness?
The phrase “real photo” is incoherent. It makes sense not as a description of an image’s ontological status but only as a judgment about image makers, their techniques, and, ultimately, their intentions, as if realness requires the elimination of any particular aim or human point of view. In a sense, the “artificial intelligence” of text-to-image models does precisely that, averaging together billions of indiscriminately ingested images to arrive at statistically based representations of any articulable concept, extracting its “real” essence from empirical contingencies and subjective distortions. Yet evidence of subjectivity is what ordinarily allows us to regard an image as real: as evidence that someone saw something in some particular way and found the means to share that vision. A generative image violates our sense of reality not necessarily because it looks too much or too little like what it depicts but because it tricks us into attributing a point of view to a synthetic depiction.
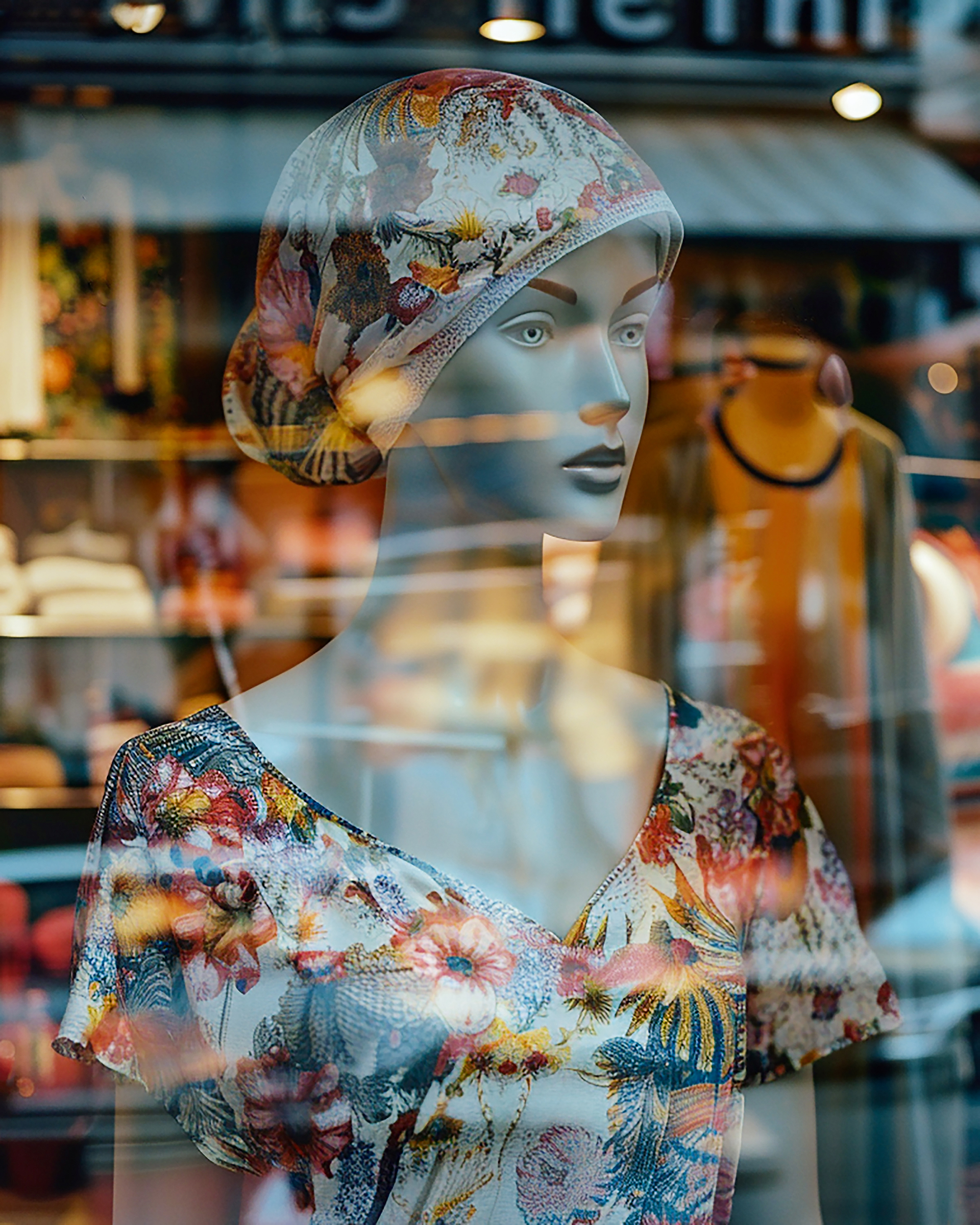
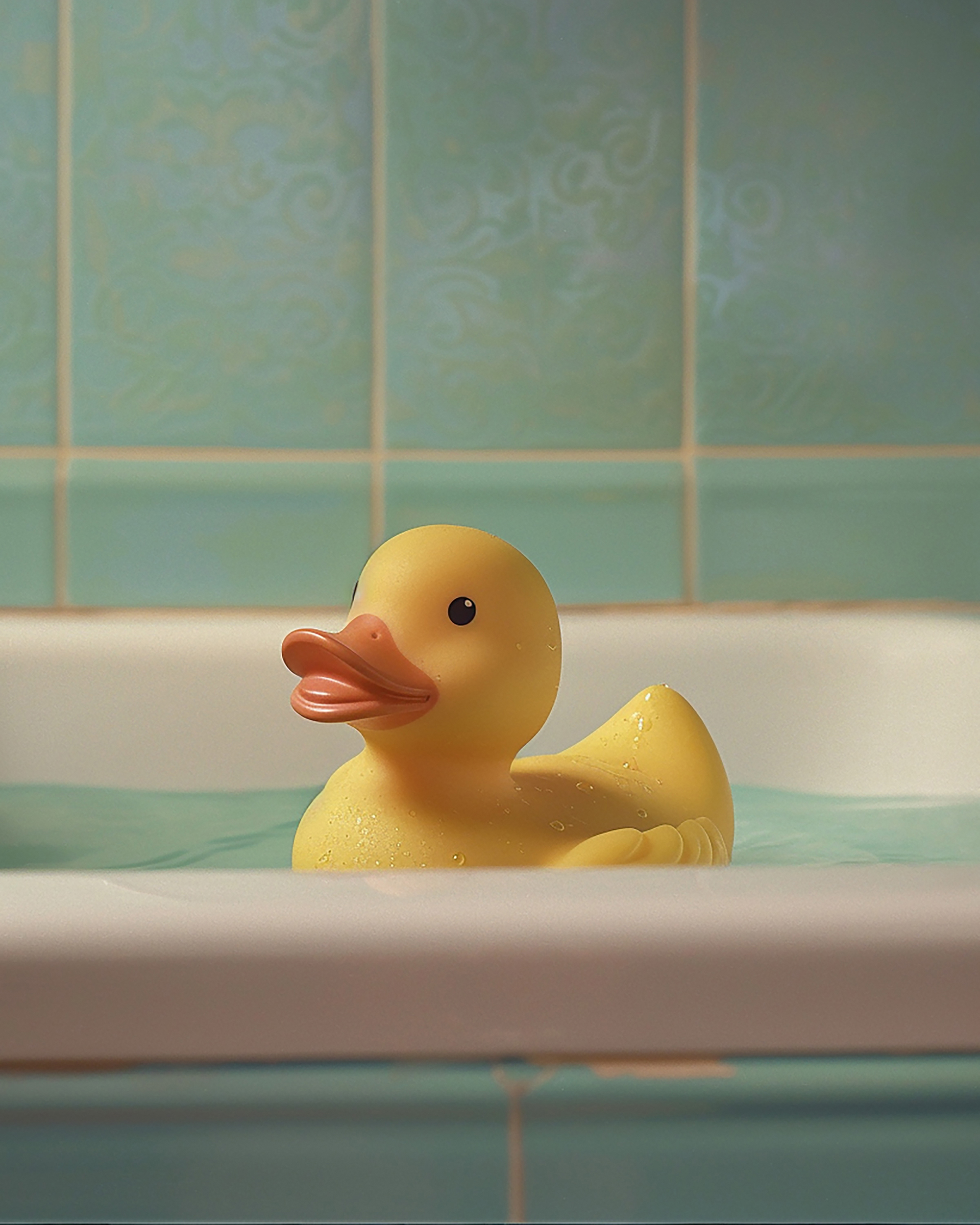
We find an increasing tension in the commonsense notion of a “real photo.” The term is now used to indicate that an image is not machine generated (rather, it is subjective), but it also retains the sense that an image is not faked in more familiar ways, not edited toward some preconceived notion or purpose (it is not subjective). Meta’s recent labeling controversy reflects this confusion in that its reality detector was calibrated to identify any form of digital editing as proof of the adulterating presence of AI, inadvertently stigmatizing retouching processes that have come to be largely accepted as non-falsifying. Conflating any kind of postproduction with automatic generation suggests how “Made with AI” has no clear, stable definition at all. “If ‘retouched’ photos are ‘Made with AI’ then that term effectively has no meaning,” the photographer Noah Kalina posted on Threads. “They might as well auto tag every photograph ‘Not a True Representation of Reality’ if they are serious about protecting people.”
Given its track record of fomenting hate and violence, abetting commercial surveillance and discrimination, and treating users as guinea pigs in undisclosed psychological experiments, it’s hard to believe that Meta is especially serious about protecting people. But even if it were, it’s not clear why such a label would be protective, or why Meta or any other for-profit company with a vested interest in how media is consumed should be regarded as a reliable custodian of reality. It seems safer to take in earnest what Kalina suggests and treat anything posted to social media as effectively falsified by its uncertain provenance and context than to feel authorized to believe everything you see unless Facebook tells you not to. To count on content labels would not only represent a sort of moral hazard with respect to media literacy (“Don’t worry, media platforms will interpret images for you”), it would suggest that the only “safe” images are those that require no interpretation in the first place. This treats users’ interest in images as being strictly a matter of expediently extracting information from them; the best images from this standpoint are verified as “real” in a glance and transfer their data load as efficiently as a hypodermic needle. That way you can consume more, faster.
Aperture Magazine Subscription
0.00
A tech and media industry group called the Content Authenticity Initiative criticized Meta’s implementation, arguing that only “wholly AI generated” images should be labeled “Made with AI.” But this approach ignores the crux of the problem: the complexities of distinguishing what is “generated” and what is “manipulated” as automated software tools do more to blur the two together. Far from initiating authentic content, the group’s proposition uses the threat of AI to try to bolster the privileged relation to reality of photographic techniques that can’t support it and media outlets that categorically don’t deserve it. Moreover, such labeling figures viewers as bad information processors—who expect images to be dichotomized into true and false but can no longer count on their faulty sensors—and marginalizes the value of ambiguity, irresolution, multivalence, and other aesthetic modes of engaging with images.
“Made with AI” labels presume that, normally, anyone can tell which photographs are “real” simply by looking at them, without any context indicating why the image was made or circulated. This is the implicit theme of a quiz that the New York Times published on its website, in June 2024, headlined “A.I. Is Getting Better Fast. Can You Tell What’s Real Now?” The quiz shows a series of images of celebrities and politicians with a few evocative wild cards mixed in, some of which are machine generated. Echoing the well-rehearsed line of fearmongering about deepfakes, the accompanying copy insists that “fake images can increase the risk that people will be deceived online, and they also risk eroding the public’s trust, making it harder to believe genuine images.” The overall implication is that if you mistakenly believe that a picture of Dwayne “the Rock” Johnson wearing a police uniform in a mall is “real,” that proves how AI has overwhelmed your feeble epistemic capabilities and become a threat to reality as we once knew it.
But if machine-generated images are hard to distinguish from “real” images, it might tell us less about the power of AI models and more about how media contexts have always conditioned our understanding of what we see, patterning “reality” in familiar, formulaic ways. Even if one could spot reality in the surface of an image, as the New York Times’s test implies one should, it would remain a meaningless skill in the abstract and afford no protection, let alone gratification. It’s not as though audiences, even news consumers, only ever want to feast their eyes on facts. Spotting fakes under laboratory conditions says nothing about how well one grasps the “rhetoric of the image” (to borrow a phrase from Roland Barthes) and runs counter to much of the pleasure that looking at images affords.
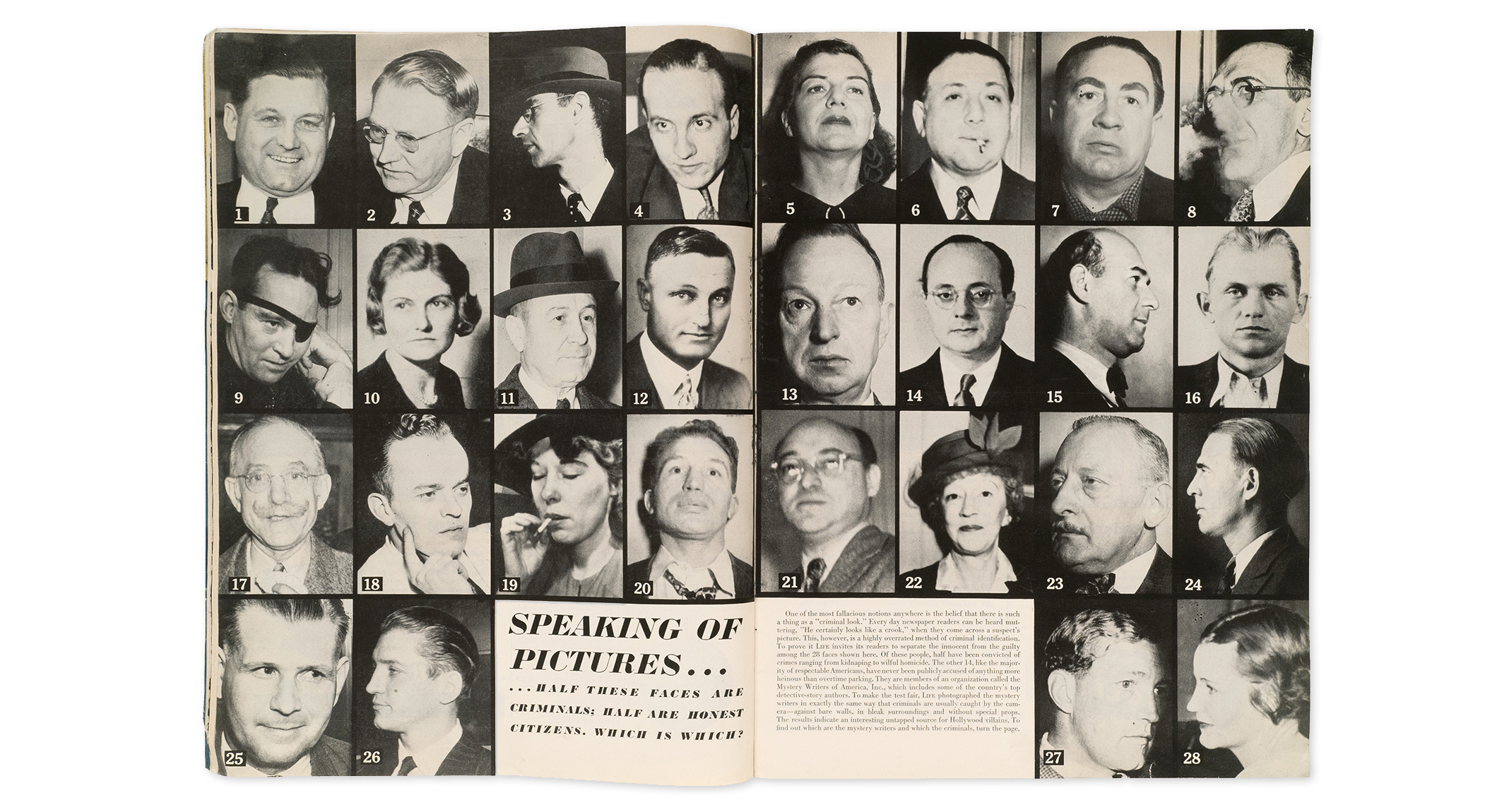
Spread from Life magazine, 1947
The New York Times quiz is reminiscent of a 1947 Life magazine quiz—mentioned by Fred Ritchin in his 1990 book In Our Own Image: The Coming Revolution in Photography, an early attempt to reckon with digital imaging’s impact on documentation—in which readers were shown a series of headshots and invited to decide which were of criminals and which were of mystery writers. The ostensible point was to disabuse readers of their physiognomic biases. “It was a seductive test, quasi-scientific in its appearance,” Ritchin writes, yet the photographs were “cleverly selected” to trip readers up, playing to and implicitly reinforcing visual stereotypes. By using images of writers with the lighting and camera angles of mug shots, the Life editors hoped to trick readers into drawing conclusions that would make them question their ability to accurately understand the world. As Ritchin puts it, “Life, purportedly challenging stereotypes, primarily managed to show the magazine’s talent in directing the reader.”
To care about which images in the New York Times quiz are faked is not to care about reality as such but about the capability of the newspaper to dictate it, the ability to pass off the photographs it selects for editorial impact as also being reputable presentations of truth. Its images, too, are, in effect, generated, presenting a concept in a heightened, exaggerated, or purified form. The catastrophizing over inane but by and large harmless phenomena such as AI-generated “shrimp Jesus” images on Facebook (which are pretty much what that phrase would lead you to expect) works to recuperate the more established forms of staged imagery in conventional media. It would suit both traditional media’s and social media’s interests if it became customary to assume any image was “inauthentic” unless validated by their imprimatur. Then only someone with press credentials or a platform’s BlueCheck verification could publish a “real photo.”
Deep reals are what happen when documented reality has the grammar of fakeness.
Published just as digital photography was beginning to become pervasive, In Our Own Image suggests that the pleasure of consuming photography was still bound up with its automatic visual realism. “Scenes photographed in a straightforward way are presumed to have contained the people and objects depicted,” Ritchin writes. “Unless obviously montaged or otherwise manipulated, the photographic attraction resides in a visceral sense that the image mirrors palpable realities.” Audiences, in his view, were unprepared for a shift to “photographic simulations.” He adds, “Should photography’s relationship to physical existence become suddenly tenuous, its vocabulary would be transformed and its system of representation would have to be reconsidered.”
That reconsideration is underway. No one now could be ignorant of how photographs are manipulated and montaged; nearly everyone has engaged in such manipulation themselves on their phones. And generative models’ capacity to synthesize and augment images has been widely, relentlessly publicized, with ostensible warnings such as Meta’s “Made with AI” labels adding to the hype. But the reconsideration isn’t one-sided: As people have become more fluent in expressing themselves with images and more familiar with the ubiquity of digital manipulation, they have not correspondingly become more cynical about how images can deceive. They also enjoy what images can be made to say, the concepts they can clarify, the social bonds they can help sustain. Viewers don’t necessarily approach images expecting visual transcripts of reality, but neither have they become fully disillusioned of photography’s promise to preserve a moment. Savviness and disavowal proceed together.
Audiences, of course, still find some “photographic attraction” in realism, but that desire for realism should not be confused with a hunger for evidence. The kinds of images that circulate the widest, especially now, are not the “most real” but those optimized for attention. However, deepfakes don’t call out for deep attention; they want to compress into a superficial glance the desire to look. A deepfake with malicious intent relies on the same logic as content labeling: that viewers approach images as if they are absolutely true or false and decide instantly which is the case. It expects to be seen as true at a glance, injecting its false information into the hapless, duped viewer. Generative images that hurry viewers from idea to already-rendered output, and editorial photography with its reinforced stereotypes, work similarly, aiming at a realism that confirms biases, passing them off as what everyone else already thinks.
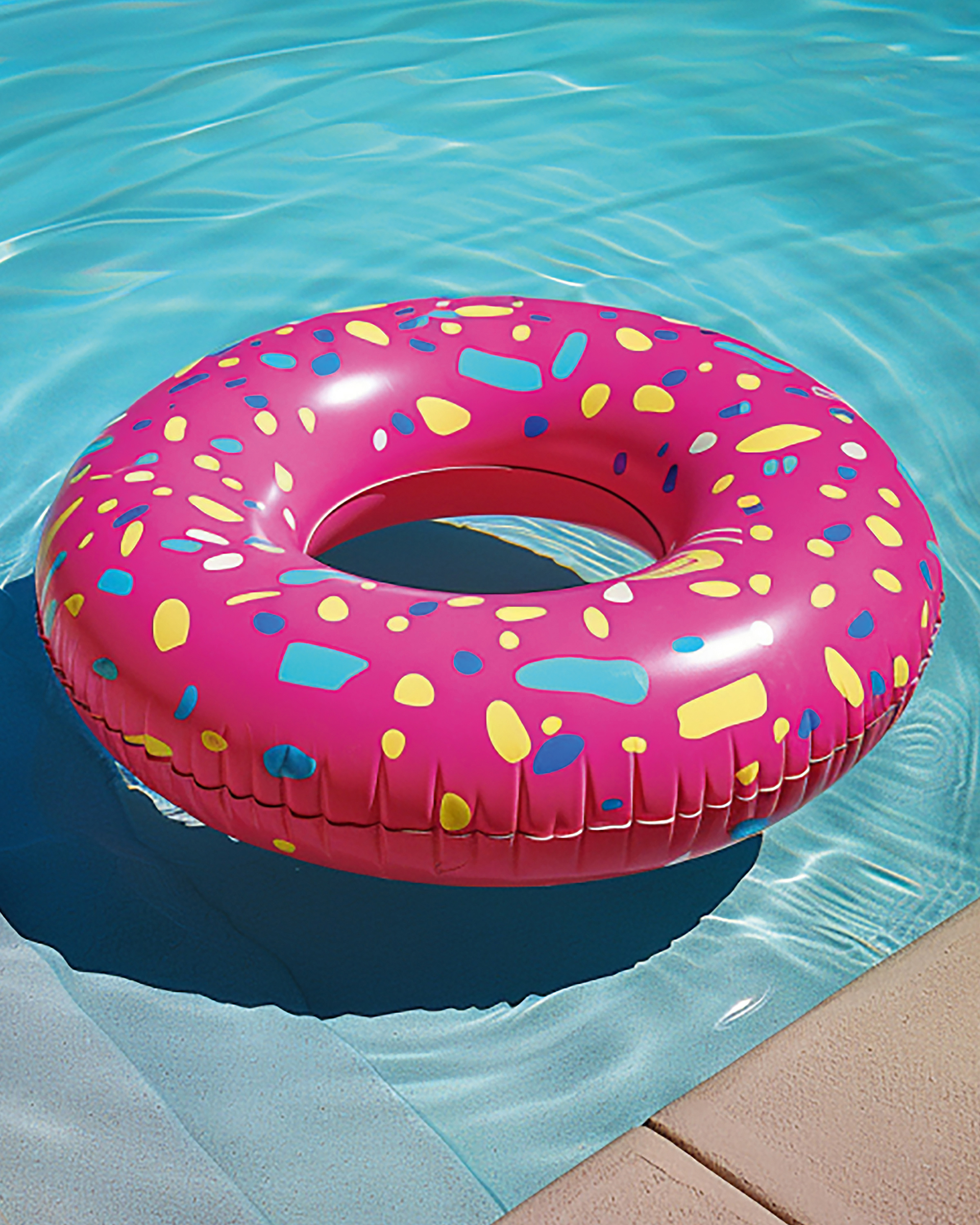
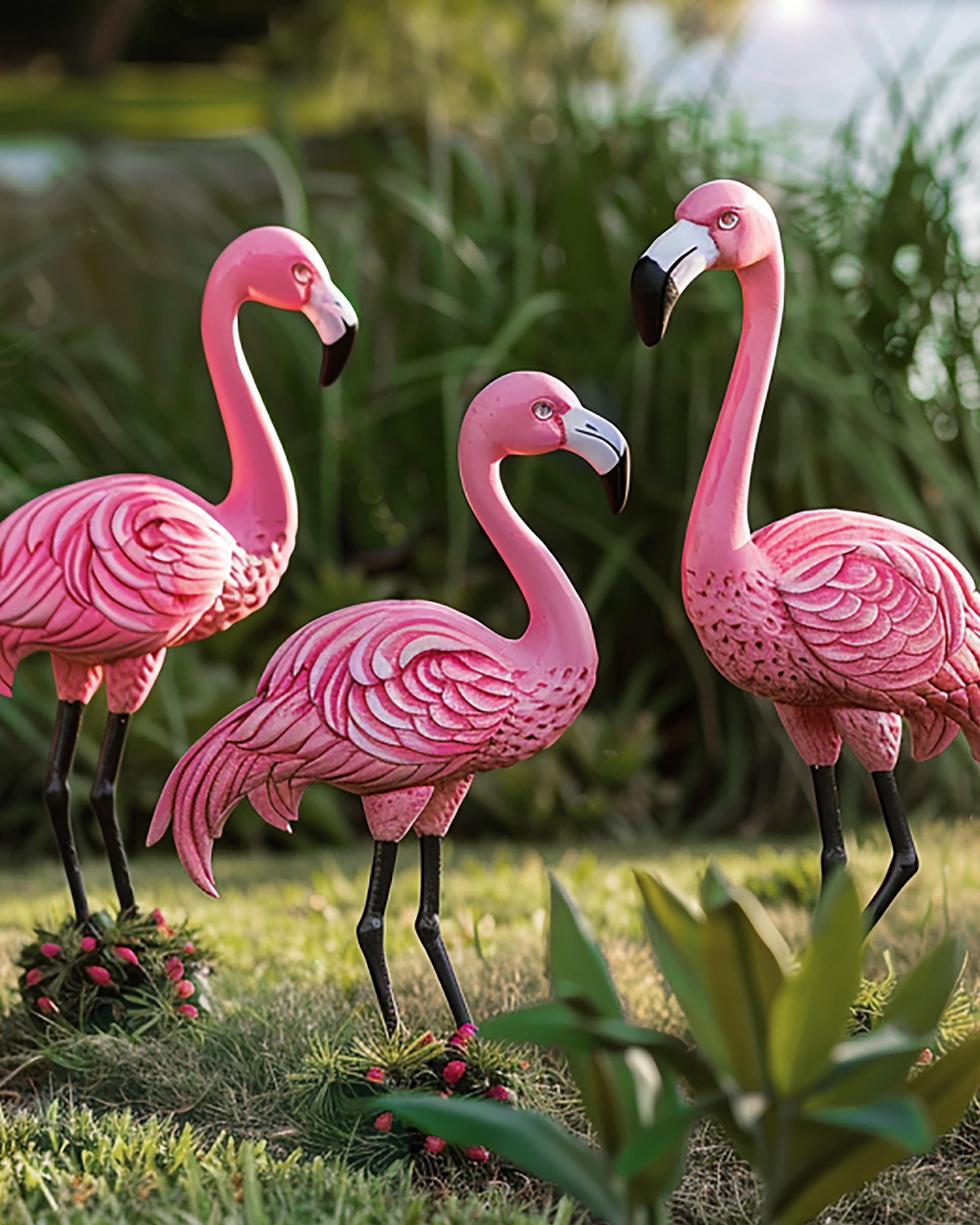
What is experienced as real—what seems “authentic”—derives from persuasive details, not accurate ones. Realism is not a mode of documentation, a degree of fidelity, but a set of conventions that coalesce around an affective appeal, a kind of nostalgia for a shared ground truth, “what everyone believes,” rather than an irrefutable depiction of it. A deepfake plays to the idea that what can be made viral will seem more and more real until it achieves a kind of Swift boat–esque certitude from being repeated and reported so often. The image’s notoriety becomes its main context, if not the “real” thing that’s captured, overriding what is represented in the image. The notion of “realism” becomes a participatory mode of consumption. The reality inheres in the circulation and not the event.
But as our relation to realism is affective, it is not resolved through assigning pass/fail grades to images on a reality test. Images that suspend us between the two, or between different interpretations, can be more compelling, more participatory, and go as viral (as the arguments over what color “the dress” was in 2015 demonstrated). Enjoying “realism” is not just seizing a moment of validation and authentication, it is also being suspended between the real and the possible.
One might call such images “deep reals,” a phrase that the social theorist Nathan Jurgenson used to describe videos taken during a recent tornado outbreak in Nebraska. For many people, the twisters in these clips seemed too realistic to be real—too much like what an image generator would produce. The instantly iconic image of Donald Trump after the attempt to assassinate him is another example. Deep reals are what happen when documented reality has the grammar of fakeness, of computer-generated image simulation. It is a kind of realness that depends on and plays off the ubiquity of generated images and generalized anxiety about deepfakes. They correspond to expedited visual consumption too well; they are “real” images that foreground special effects that haven’t actually been used.
Such images don’t make the preconceived and often deeply desired notions about the world disappear into a taken-for-granted visuality; they instead insist on an image’s formal qualities as being in a kind of tension with its content through their very suitability or obviousness. Rather than trying to repress our skepticism, as a deepfake would, a deep real elicits that skepticism at a deeper level, with the picture’s immediate potency undermining rather than confirming its veracity. When a deepfake presents an idea, it deliberately conceals the use of techniques of manipulation and persuasion, trying to thereby appear as a “real photo.” Whereas when a deep real gives us a ready-made concept, it foregrounds what should be taken as manipulative, challenging us to see it anew.
This article originally appeared in Aperture No. 257, “Image Worlds to Come: Photography & AI.”